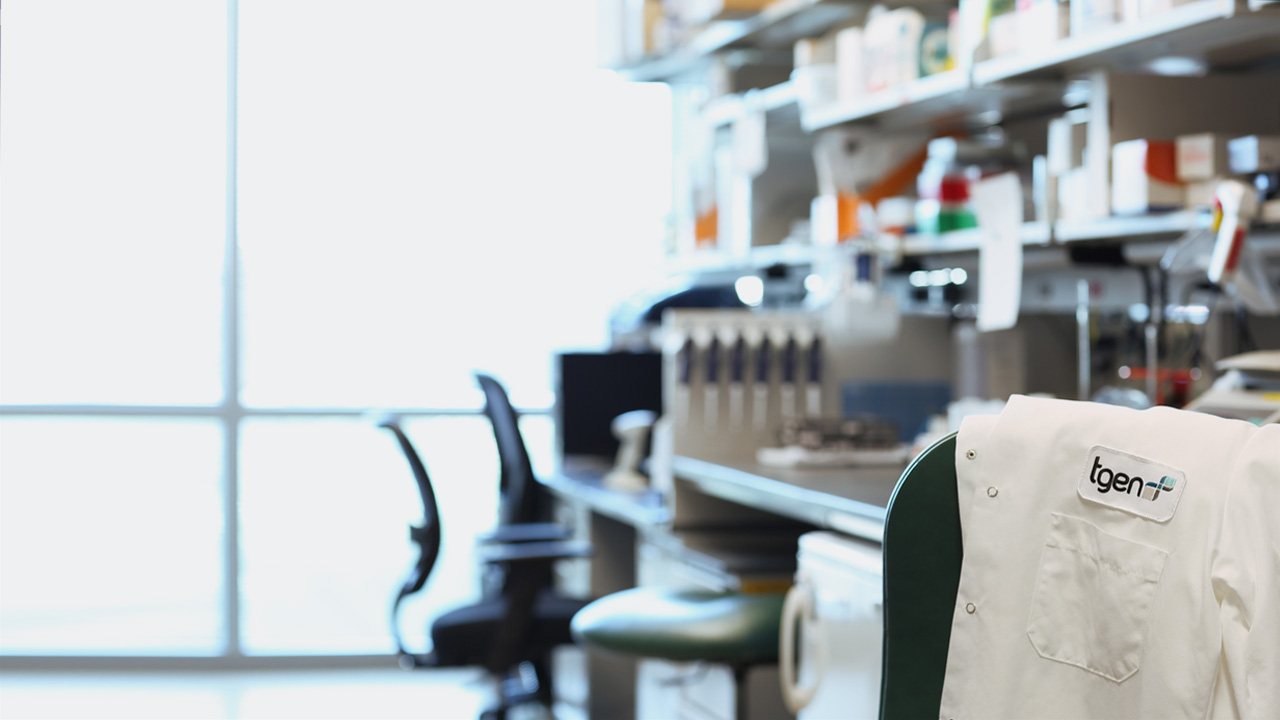
TGen, University of Wisconsin researchers use machine learning to make early detection of cancer more accessible
Phoenix, AZ — Jan. 13, 2023 — A team of researchers including faculty at the Translational Genomics Research Institute (TGen), part of City of Hope, published a study that combines genomics and machine learning in the quest to make early detection of cancer more affordable and widely available. The study was led by Muhammed Murtaza, Ph.D., professor of surgery at the University of Wisconsin–Madison.
For many types of cancer, early detection can lead to better outcomes for patients. While scientists are developing new blood tests that analyze DNA to aid in earlier detection, these new technologies have limitations including cost and sensitivity.
Published this week in Science Translational Medicine, researchers used a machine-learning model to identify DNA fragments in blood plasma shed by cancer cells. The technique uses readily available lab materials, and it detected cancers at an early stage among nearly all of the samples they studied.
“We’re incredibly excited to discover that early detection and monitoring of multiple cancer types are potentially feasible using such a cost-effective approach,” says Murtaza.
The approach hinges on analyzing fragments of cell-free DNA. Such fragments are commonly found in plasma, which is the liquid portion of blood. The fragments of genetic material typically come from blood cells that die as part of the body’s natural processes, but they can also be shed by cancer cells.
The research team hypothesized that DNA fragments from cancer cells might differ from healthy cell fragments in terms of where the DNA strands break, and which DNA building blocks (nucleotides) surround the breaking points.
Using a technique they’ve dubbed GALYFRE (from Genome-wide AnaLYsis of FRagment Ends), the team analyzed cell-free DNA from 521 samples and sequencing data from an additional 2,147 samples, including healthy individuals and patients with 11 different cancer types.
From these analyses, they developed a measure reflecting the proportion of cancer-derived DNA molecules present in a sample. They called this information-weighted fraction of aberrant fragments.
They used this measure, along with information on the DNA sequences surrounding fragment breaking points, to develop a machine-learning model that would compare DNA fragments from healthy cells to those from different types of cancer cells.
The model accurately distinguished people with any stage of cancer from healthy individuals 91% of the time. In addition, the model accurately identified samples from patients with stage 1 cancer in 87% of cases, suggesting it holds promise for detecting cancer in early stages.
The information-weighted fraction of aberrant fragments method is “shown suitable to detect changes in tumor burden over time in confounding brain tumors like glioblastoma, which could also offer real-time efficacy assessment of ongoing treatment of this aggressive disease,” says Michael Berens, Ph.D., Professor and Director of the Cancer and Cell Biology Division at TGen and contributing author on the paper.
Murtaza says that while the current results are promising, more studies are needed to refine GALYFRE’s use in different age groups and when patients have additional medical conditions. The team is also planning larger clinical studies to validate the test for specific cancer types such as pancreatic cancer and breast cancer.
“One direction we are taking is refining GALYFRE to make it even more accurate for some patients who are at risk of developing specific types of cancers. Another aspect we are working on is determining if our approach can be used to monitor treatment response in cancer patients who are receiving chemotherapy.”
“My hope,” says Murtaza, “is that with additional development, this work will lead to a blood test for cancer detection and monitoring that will be available clinically in the next 2-5 years for at least some conditions, and ultimately be accessible for patients with limited healthcare resources in the U.S. and around the world.”
More news from the PBC
- PBC Arts Committee Student Grant to showcase student research and innovation through art
- Ten Arizona research teams earn $100K Flinn Foundation seed grants
- Unlocking the immune system’s potential to fight skin cancer
- ASU professor named Rural Health Fellow
- Phoenix Bioscience Core Unveils XLR8 PBC to Propel Scale-Ready Health Tech Companies
- ASU selected as home and partner for CHIPS and Science Act-funded national facility for semiconductor advanced packaging
- $3.9 Million Grant Seeks to Expand Primary Care and Rural Tracks for Medical Student Training in Arizona